Posted by Dr Catrina Denvir, director of the Legal Innovation Centre at the University of Ulster
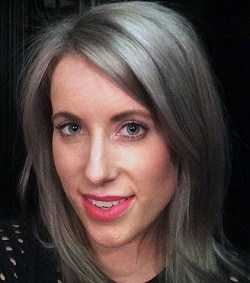
Denvir: we need to reach ‘second order change’
Over the last decade, a growing group of ‘futurists’ have declared the lawyer an endangered species.
Drawing on the disruption other industries have faced as a result of technology, these commentators have issued stark warnings as to the future of the legal profession.
Whilst proselytising about legal technology can help prepare the profession for emerging trends, changes and their likely impact, it is difficult to convince a lawyer that a particular proposition is correct by relying on anecdote alone.
If the legal profession is not yet on board with the idea that we are undergoing substantial change in the profession, it may have less to do with the vigour with which the message is conveyed and more to do with what is being said, which, all too often is nothing of any real substance.
Lawyers have long been depicted as a cartel of self-interested obstructive technophobes; but if it was ever the case that those in the legal profession are Luddites, it certainly no longer remains so.
No modern lawyer would pick up a quill if they could download a precedent, and no corporate client would let them. Within the legal industry, the market has dictated the price at which certain legal services will be set and the legal service provider now has to determine how they can meet this price and maximise the gains.
It is hardly revolutionary that clients will not tolerate receiving hourly billings from a law graduate with a 20wpm typing speed. The practice of law has become more transparent and most clients can identify bad value. They also now have the means by which to address this bad value – a large, competitive legal marketplace.
Whether it is near-shoring or service centres, outsourcing to the Asia-Pacific or introducing greater automation, technology will play an increasing role in maintaining the profit margins of those who provide legal services. However, the adoption of technology as a means by which to make existing processes more efficient is not transformational – at least not in the way that the futurists purport.
Automation has largely been deployed within the confines of existing practice approaches. Legal start-ups are often the brainchild of a lawyer who has had a particular ‘pain point’ during their practice and has built an offering around that.
Piecemeal automation breeds a proliferation of ad-hoc tools used for specific parts of a broader legal task/process: Ravn for due diligence or contract analysis, Contract Express for document automation, Dealroom for M&A process management.
Thus, we find ourselves, technologically speaking, at what Gregory Bateson terms the stage of ‘first order change’ – change that integrates into existing structures, is reversible, non-transformational, requires little new learning and restores the old balance (of profitability) without too much adaptation or behavioural adjustment.
That the legal profession is not concerned by the implications of such change is hardly surprising. When AI is used as a marketing tool for any and all software that employs even a modicum of AI-related technology such as machine learning or natural language processing algorithms, disappointment is inevitable. Lawyers may well question the threat that such technology presents in its current form.
What, then, is needed to bring about the second order change Bateson characterises as heralding irreversible modification of the practice of law and a ground-up reconsideration of how work is organised, undertaken and executed? The answer isn’t just more automation, but rather, technology of a different type.
Setting aside the humanistic elements of the job, the work of a lawyer is the management of information and knowledge relating to a number of domains: a client’s goals, the law, processes, procedure, the contents of key documents, or of fact.
Specialised knowledge is the defining feature of all professions and the extent to which a profession is exposed to technological disruption depends on a number of factors, including the level of manual dexterity involved in a task and the nature of the data handled (linguistic or numeric).
Manual dexterity is relevant because the motions of the human hand are difficult to replicate. This is one of the reasons why, although medical practice has and will continue to be influenced by intelligent diagnostic systems, the skills of a surgeon will continue to be necessary until robotics have advanced to such a state that they can replicate this movement.
Compare this with finance-related professions where dexterity is largely irrelevant, knowledge is stored as numbers and expertise is dependent on one’s ability to compute and detect patterns in this numeric data. Computers can perform these calculations/analysis better, quicker and more accurately than a human ever could, leading to great upheaval. If you are a broker, there is nowhere to hide if a computer can execute buy/sell decisions more accurately and reliably than you can.
Law, whilst requiring a low level of manual dexterity, has been somewhat insulated from disruption due to the fact that legal knowledge is represented semantically (the language of humans) rather than numerically (the language of machines).
For example, suggesting a likely interpretation of a section of legislation requires an understanding of context, knowledge of existing law and precedent, and a sense of the logic or reasoning employed by a judge.
Given enough data, an intelligent system could arrive at a mathematical prediction of the likely interpretation of a section of legislation based on the inputted data. However, in order to do so, the system would need to be told what features to look for in the data. Further, these features need to be encoded in a language that such a system could actually understand.
This is a difficult task that requires lengthy periods of development and finesse to ensure accuracy. Further, it relies on bringing together advances in a number of AI domains, notably natural language processing, machine learning and automatic taxonomy/ontology construction. However, this is no longer a distant prospect thanks to the surge of interest and research in machine learning and neural networks over the last decade.
Machine learning (often used interchangeably with the term ‘artificial intelligence’) presents us with fundamental advantages when it comes to dealing with the law computationally. Taking in large amounts of (unstructured, non-numeric) data, machine learning can solve tasks that are difficult to solve using traditional rule-based programming approaches. This applies to both the identification and auto-encoding of relevant variables from a large set of data (eg, legislation) as well as then determining how to apply that encoded data.
Machine learning allows us to create a machine that can learn to do something in an unsupervised fashion, rather than programming a machine to do something. It does not require that a human intermediary identify all the factors to extract, or the steps for a computer to undertake, but rather, lets the system figure these issues out.
Not only does this have application across a wide range of knowledge domains (law is just one of many subject areas to which this technology is applicable), but it also brings us closer to replicating some of the features of the human brain, albeit in a different way.
The human brain is distinct, partly because of its ability to take knowledge gathered in one setting and apply it to another setting. If I ask you to imagine running down a hill with a bucket of water, you do not need to have previously run down a hill with a bucket of water before to know what is likely to happen – the water will slosh onto the ground. This type of transferability is hard to replicate in computers, although it is fundamental to the process of learning.
Machine learning (through neural networks, for example) can achieve this by building complex concepts out of simpler concepts, allowing, in effect, knowledge acquired in relation to one task or one part of a task, to come together in the resolution of another task.
Up until 2016, Google Translate used phrase-based translation, the equivalent of looking up words in the dictionary. It is a reasonably accurate tool, but can be somewhat crude in failing to understand words in the context of a sentence.
In September 2016, the company adopted a new approach to translation, building the Google Neural Machine Translation system to utilise neural networks and machine learning to undertake translation. Very quickly, it got quite smart, detecting the likely interpretation of a word based on the context of the words surrounding it and adjusting its approach based on user interaction.
More interestingly, it independently identified ‘interlingua’ (a broad term for the universal rules underlying language), which enabled it to translate between languages it had not been programmed to translate between. So whilst trained to translate between (i) Japanese and English and (ii) English and Korean, the system figured out how to translate between Japanese and Korean through the common language of English.
In so doing, it demonstrated the potential for machine-learning algorithms to achieve ‘one-shot learning’: to perform a new, unfamiliar task, by drawing on knowledge in a related domain without needing vast amounts of training data.
Developments such as this reinforce the argument that we need to move away from using technology to try and recreate the thought-process of a lawyer. The idea is not to build a lawyer’s brain, although we may well want to approximate some of its features.
Rather, the potential tech offers is in designing a system that arrives at a similar, if not a more accurate or informed outcome as a lawyer might, by building on the strengths that computers have that humans do not, namely: the ability to simultaneously process large amounts of data, to calculate probabilities more accurately, and to identify patterns and relationships that exist across a large evidence base.
We must move beyond the combative idea that we are trying to create a robot lawyer to replace lawyers. The future of law requires us to reflect on how we might embody the concept of a robot lawyer ourselves, using technology symbiotically to both complement our human skills and compensate for our human shortcomings.
Follow Catrina Denvir on Twitter here.
That’s a whole lot of words without mentioning ROSS, an AI tech already in big firms, including Dentons. Google it.