A guest post by Ken Grady, the Lean Law Evangelist for US law firm Seyfarth Shaw
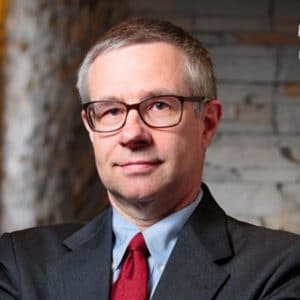
Grady: lawyers are not engaging with the issues raised by emerging technologies
I recently made my predictions for 2017, and one was that pundits and others in the legal industry would keep talking about artificial intelligence (AI) and law. Since I want to get 100% on my predictions, again, I thought I would start the New Year by ensuring I at least got this one right. So, I’ll talk about AI and law.
I am going to skip the usual topics when AI and law comes up: when will LawNet go live; will Arnold Schwarzenegger agree to play Chief Justice of the Future in the mash-up of Terminator and First Monday in October. Instead, I am going to focus on some questions that you do not hear discussed every day. They circle around an interesting question: are the emerging technologies, such as AI and smart contracts, about to make law more brittle?
To understand where I am going, you need a bit of a running start. First, AI. AI in law is based on machine learning (outside law as well, but let’s not go there). In very simple form, using machine-learning tools, data scientists have computers hunt for patterns.
Given the power of computers, they can hunt for patterns where humans would never find them. A computer can ‘watch’ millions of videos of cats and find patterns that it can use to define ‘cat’. Show the computer a new group of videos, some with cats and some without, and the computer will do a very good job of separating the cat videos from the non-cat videos. Sounds a bit like separating relevant from irrelevant documents in discovery, doesn’t it?
Let’s try that same trick with US Supreme Court cases. First, understanding the text of a case is much more difficult than understand ‘cat’ from ‘non-cat’. Second, the data set to learn about cases is much smaller than the data set to learn about cats. The Supreme Court has issued fewer than 30,000 decisions on the merits. Compare that number to the volume of other stuff out there:
- 300 hours of YouTube videos are uploaded every minute (that’s right, 432,000 hours each day);
- 9 million blog posts are published each day on WordPress alone; and
- Over one million books are published each year.
When it comes to data sets, the volume of material ‘available’ for a computer to chew on in the law is minuscule compared to the volume of materials computers outside the law use to learn about the world.
I put ‘available’ in quotes because much of law is not available. It is locked behind absurd paywalls or in confidential files. Much of it is not digital or is barely digital.
The small volume has meaning. The lower the volume, the harder it is for the computer to find patterns unless they are incredibly obvious. The Supreme Court decisions are already a small data set, but we have to further break that down. The cases are not all on one issue (like cat videos all contain cats), so a computer attempting to learn bankruptcy, antitrust, or securities law has far fewer decisions to chew on.
And, of course, the cases in any substantive domain—say, securities law—don’t all cover the same issue. The computer is not looking at 1,000 cases on the standard for liability under rule 10-b(5) (a Securities & Exchange Commission prohibition on fraud), it is looking at one case. Some of the cases do overlap and the court does come back to issues, but the variability in case law is tremendous. ‘Cat’ also is variable, but when you get to look at millions of cat videos, it is much easier to find similarities than when you get to look at just a few cases.
Let’s assume we throw in all the federal cases on rule 10-b(5) (at one time, the dean of my law school kept a copy of every 10-b(5) decision and had the cases in file cabinets outside his office), so the computer has a larger data set, though still small by most standards. The computer chews on these cases for a while and finds what we will call some rule 10-b(5) patterns.
Our idea is to apply these patterns to new fact situations, and let the computer predict possible outcomes. For example, we might ask the computer, ‘What are the odds that we will win this case if we go to trial and file any necessary appeals?’ The computer considers our fact pattern and replies:
- 60% probability of a ‘win’ at trial;
- 35% probability of a ‘win’ at the federal appellate court; and
- 5% probability of a ‘win’ at the Supreme Court.
I’ve glossed over many things to get us to this point, so don’t think we can do this today or that our data sets are up to the challenge. Just assume with me that we could get these answers.
This is where the brittle problem comes in to play. The computer can only learn from looking at the text of the cases. To put it in Donald Rumsfeldian terms: the computer knows what it knows, but it doesn’t know what it doesn’t know. The computer cannot consider what the judges in those cases may have considered, but never wrote in their decisions. The problem reminds me of an exchange I once heard in a deposition:
Did Tom attend the meeting?
A. I don’t recall.
Q. Did Dick attend the meeting?
A. I don’t recall.
Q. Did Harry attend the meeting?
A. I don’t recall.
Q. Well, who was at the meeting?
A. I don’t recall.
Q. Well then, who wasn’t at the meeting?
A. Uh, well, most the people in the world, I think.
The computer does not know what the judge considered that was in the record (presumably a data set that would be possible, if difficult, to create) and certainly does not know what the judge considered beyond the record (did the judge do some Internet research, rely on his priors, or perhaps gather information through discussions with others about hypotheticals?).
The AI assumes that what it reads is the truth. If the judge says that facts X, Y, and Z form the basis for his opinion, then the computer assumes they did indeed form the basis for the opinion.
In reality, of course, the judge may have made up his mind and then asked his clerk to find things to include in the opinion which could plausibly add support. A human can apply scepticism when reading the decision, where the computer cannot. We do this all the time.
A Supreme Court decision holds 5-4 in favour of the appellant. We read the decision, but we know that holding for the respondent would have gone against popular opinion and caused problems for the court. Nothing in the decision hints at that problem, but it would be foolish to believe otherwise. The court is a political institution. The reasoning in the decision sounds plausible, but few believe that reasoning tells the real story of the decision.
Depending on how the AI analysis is used, it can make the law brittle. It gives the users the appearance of mathematical precision (60% probability), in part because it has difficulty sorting between what is known and what isn’t known. Over time, layering AI analysis upon AI analysis can lead to cases not reaching the courts that, had they made it, would have built additional factors into legal decisions and kept the law plastic.
Smart contracts or brittle law
Now let’s look at smart contracts. One premise behind smart contracts is that we can code into the blockchains ‘if-then’ situations, leading to predictability and certainty in outcomes.
If I make a deposit into your bank account in an amount equal to X on or before a certain date, then you will record my payment as complete. If I do not make the payment on or before the date, or if the payment is less than X, then you will record my payment as incomplete. If my payment is incomplete, you will declare my account in default.
Today, we have computers that follow this process and, if the payment is incomplete, generate an exception report. The exception report may trigger a letter ‘We have not received your payment’ or something harsher – ‘Because you failed to pay on time, we have closed your account.’
I call and explain that you mailed the cheque on time, but it arrived later than the due date. I invoke the ‘mailbox rule’ (payment was complete when I deposited the check in the US Mail) and you relent and mark my account as ‘current’ (since you received the payment).
We can live with plastic law (which we call equity) and modify the outcome based on the circumstances. Or, we can move to brittle law—the outcome depends on the ‘if-then’ statements. Once we say the outcome may depend on the if-then statements, but equity (humans) will get involved when there are exceptions, we move from smart contracts back to our current world. In other words, we raise the question: how brittle do we want to make the law?
There is, of course, a trade-off. We can keep the law as plastic as it is today, but use smart contracts as a way to replace some of the cumbersome and not very secure aspects of our current systems. In other words, we don’t use smart contracts to make the law more predictable, but we do use them to make the transactions more secure.
The registry for chain of title is put on a blockchain, so we can all view it and rely on it. But, if there was an erroneous entry in the blockchain, humans will consider it and update the registry (put a new entry in the blockchain), where there was an error.
I have given a simple description of smart contracts to demonstrate a point, and there is a lot of grey area I did not cover. That grey area represents the many issues we should address as AI and smart contracts move into law. It represents the bigger question of how technology and humans should work together.
Lawyers should shape, not fight, the future
If I did my job, this essay raises many more questions than it answers. That is good. Emerging technologies, such as AI and smart contracts, are raising lots of issues. Our problem is not that there are issues, our problem is that lawyers are not engaging with the issues and working on answers.
We are heading down a path where technologists move law from the current structures onto digital platforms. But, we haven’t thought through the consequences. We will never know all the consequences in advance, but I think it is fair to say today we have put very little effort into thinking through the consequences so we are far behind the curve on helping enable a successful integration of these technologies.
Most of the chatter about AI and smart contracts is of the ‘What about me?’ variety. Will AI take my job? Will smart contracts eliminate the need for lawyers?
We should instead focus on the applications and implications of these technologies and do what lawyers should do: consider how to make them work in our society and raise flags where we see conflicts and problems.
Putting a drag on the system because we are afraid of or do not understand the technologies does not help anyone, including lawyers. We should start off 2017 by looking at how we can help society, not just how we can protect lawyers.
This blog first appeared on the blog of SeyfarthLean Consulting.
Leave a Comment